AI-powered recruitment is programmed to capture to measure diversity data regarding gender and race, explicitly using it in algorithm design. Yet these are the very things to avoid in terms of preventing race and gender bias, says Was Rahman
Artificial Intelligence (AI) is an established feature of recruitment, having become an intrinsic part of software that large employers and agencies use through the hiring process. It’s therefore become important for employers to understand what’s behind periodic headlines about apparent racism or sexism in AI, and whether these are simply teething problems with new technology, or something more significant.
We’re going to explore two important factors involved. One relates to how AI uses data about existing employees, the other is about potential AI implications of the very HR policies intended to address potential racism or sexism in recruitment.
Of course there are several others, and even these two are complex to understand fully. But awareness of these in particular is a minimum starting point for recruiters today.
What recruiters use AI for, and why
AI in recruitment primarily supports or replaces traditional human activities, rather than doing something new that recruiters haven’t usually done before. This is typically sourcing, screening, assessing and engaging with candidates, and the main benefits sought are around efficiency, scale and cost. Candidate engagement AI is similar to customer service technology such as chatbots and automated correspondence and is not an area where bias issues appear often, so we’re focusing on AI in applicant sourcing, screening and assessment.
AI has not just reached these functions through standalone AI systems, but often by adding AI features to core recruitment systems. This is similar to other business processes such as sales and churn management, where a common way of using AI to improve them is through new AI features in existing CRM systems.
The recruitment equivalents are ATS (application tracking systems) and online job posting, which provide natural opportunities to apply AI. As a result, employers can process far more applications than previously possible, and at significantly lower cost. To understand how this can lead to racism and sexism in recruitment, we should look at how such AI works, both generally and in recruitment systems specifically.
It’s worth noting that there has been significant progress on using separate AI applications to improve workplace diversity, but there are challenges and questions around how effective these are and how they work in practice, so we won’t be discussing that here.
How AI in recruitment works
Underneath all AI technology is computer software that analyses massive quantities of data to find patterns and draw useful conclusions from it using statistics and maths. That data may be pixels from a camera image that are compared with other images, to recognise a face. It may be comparing a viewer’s behaviour with other customers’ selections, to make movie recommendations. Or it may be digitised sounds being compared with examples of speech, to recognise words and their meaning.
AI systems use two elements to draw conclusions such as recognising a face, recommending a movie or understanding a spoken instruction: algorithms and training data.
- An algorithm is the logic that AI uses to decide what factors and statistical models to apply. For example, a set of equations and statistics to predict the likelihood of a customer liking a movie.
- Training data is a large set of data to which algorithms are applied to achieve desired results. During training, parameters are adjusted until the algorithm achieves accurate results with this data.
The training data needs to be similar to the data the AI will be fed when operational, such as images of real faces, lists of movies actual customers have previously watched or recordings of normal human speech. The volumes of training data needed are immense, for example at least hundreds of thousands of faces, ideally 10 or 100 times more.
The recruitment equivalent of finding a movie to recommend is identifying candidates suitable for open positions (sourcing/screening), then evaluating fit as more information becomes available during the application process (screening/assessment).
Recruitment algorithms attempt to mathematically replicate the logic a person uses to select and evaluate candidates by comparing features of the role against public profiles, CVs and application forms. As the hiring process continues, more sophisticated algorithms can be introduced, to use new data such as interview and psychometric tests results.
Training data is used to “teach” AI systems how to refine algorithms to improve accuracy. Recruitment training data consists of details of employees in the hiring organisation, and ideally similar data about people who would not be successful applicants. By applying algorithms to training data about employees whose performance is known, adjustments can be made until algorithms reliably identify employees, and recognise characteristics of those who do well. There are of course major questions about privacy and training data, but that’s a discussion for another time.
Introducing bias through skewed training data
Training data is an obvious source of potential bias in AI recruitment, because it is generated from real organisations, and reflects current and past employment patterns. So, if a company has an employee profile skewed towards white males, training data it provides will reflect that.
This creates a risk of training data implicitly “teaching” AI systems that being white and male are characteristics of successful employees, leading to a selection preference for more white males. This applies to any race or gender data patterns, such as some low-income sectors and roles.
This can be partly addressed by excluding data about ethnicity and gender from training data and algorithms, but there may remain other features of the data that indirectly correlate with them. For example, in a male-skewed sample, there will be few career breaks related to maternity or childcare, and so career breaks would likely not be a feature of successful employees in this training data. This might inadvertently lead to applicants who have had career breaks being rated lower by the AI algorithm, effectively creating bias against female candidates even if gender isn’t known.
There are many ways to avoid bias in training data, and it’s a well-understood part of AI design. But to ensure this is done properly, it’s crucial for employers to understand the issue, and know how to ensure even indirect bias isn’t present.
AI implications of diversity policies
The second factor we’re discussing in AI recruitment bias is the philosophical implications and potential unintended consequences of diversity policies. It’s much less understood than bias in training data and algorithm design.
It arises because policies to improve recruitment diversity – such as all-female shortlists or hiring quotas to ensure balanced race and gender distribution – are put into practice through operational processes and systems.
When these use AI, such policies need to be expressed in terms that can be implemented through data and algorithms. This may well mean capturing data about gender and race, explicitly using it in algorithm design. Yet these are the very things to avoid in terms of preventing race and gender bias.
There is no simple answer to this conundrum, and firms with such policies will need to decide how they will deal with it. We’ve only scratched the surface of how AI relates to race and gender recruitment bias, but it’s clear that effective, sustained answers are not simple. Technology plays a key role, and business leaders should ensure responsibility for such complex issues isn’t inadvertently left in the hands of technologists.
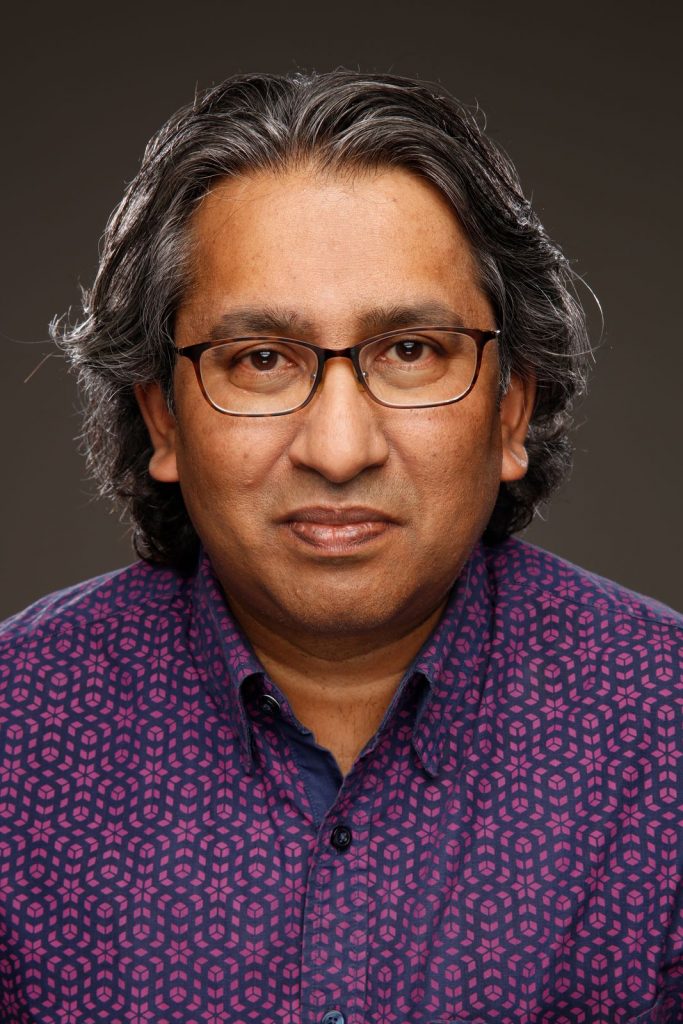
Was Rahman is an expert in the ethics of artificial intelligence, the CEO of AI Prescience and the author of AI and Machine Learning. See more at www.wasrahman.com